連續變數與離散變數
數學與統計學中,若一個定量變數通常由測量獲得,則可能是連續的;若通常由計數獲得,則可能是離散的。[1]若變數可以取兩個特定的實值,且還可以取兩者間所有實值(包括任意或無限接近的值),則變數在該區間內連續。[2]若變數可以取一個值,而其兩側各有非無窮小的間隙,其中沒有可取的值,則稱其在此值附近是離散的。[3]某些語境下,變數在數線的某些範圍內是離散的,在另一些範圍內則可以是連續的。
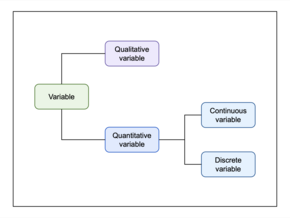
連續變數
編輯連續變數是由測量獲得值的變數,即可以在不可數集上取值的變數。
例如,在實數的非空範圍上取值的變數是連續的,因為在a與b之間的任何實數範圍 都不可數,範圍內有無限多值。[4]
統計學中,連續變數的概率分佈可用概率密度函數(PDF)表示。[6]
連續時間動力學中,時間變數被視作連續的,描述變數隨時間變化的方程是微分方程。[7]瞬時變化率是良定義的概念,是指在某特定瞬間,應變量與自變量的變化比。
離散變數
編輯相對地,若且唯若變數值與自然數集 存在一一對應關係時,才稱此變數是離散變數。[8]即,在某實值區間內的離散變數是這樣的:對可取值區間內的任何值,與最近的其他可取值都有正的最小間距相隔。離散變數的值可由計數得到,可取值數量或是有限的,或是可數無限的。常見例子是整數、非負整數、正整數或只有0、1。[9]
微積分方法用於離散變數問題不太合適,特別是在多變數微積分中,很多模型都依賴於連續性假設。[10]離散變數問題如整數規劃。
統計學中,離散變數的概率分佈可用概率質素函數(PMF)表示。[6]
離散時間動力學中,時間變數被視作離散的,描述變數隨時間變化的方程是差分方程。[11]某些離散時間動態系統的響應可通過求差分方程的解析解來模擬。
計量經濟學與更廣泛的迴歸分析中,有時一些經驗上相關的變數是0-1變數,只能取這兩個值。[12]這是為了將虛擬變數用作一個開關,將它們分配給方程中的參數來「打開」或「關閉」。若自變量是虛擬變數,則通常採用邏輯迴歸或概率單位迴歸。在迴歸分析中,虛擬變數可用於表示研究中的樣本組(如0對應對照組的組成)。[13]
混合
編輯混合多變數模型可同時包含離散與連續變數。例如,簡單的混合多變數模型可以有隻在0、1上取值的離散變數x,與連續變數y。[14]混合模型的一個例子是基於精神症狀的二元測量和認知表現的連續測量對心理失調風險的研究。[15]混合模型還可能涉及在數線的某範圍內離散,而在另一範圍內連續的單一變數。
概率論和統計學中,混合型隨機變量的累積分佈函數既不是離散的,也不是處處連續的。混合型隨機變量的例子是排隊等候時間的概率。顧客等待時間為零的可能性是離散的,而非零的等待時間是連續的。[16]
相關條目
編輯參考文獻
編輯- ^ Ali, Zulfiqar; Bhaskar, S. Bala. Basic statistical tools in research and data analysis. Indian Journal of Anaesthesia. 2016-09, 60 (9): 662–669. PMC 5037948 . doi:10.4103/0019-5049.190623 .
- ^ Kaliyadan, Feroze; Kulkarni, Vinay. Types of Variables, Descriptive Statistics, and Sample Size. Indian Dermatology Online Journal. 2019-01, 10 (1): 82–86. PMC 6362742 . PMID 30775310. doi:10.4103/idoj.IDOJ_468_18 .
- ^ K.D. Joshi, Foundations of Discrete Mathematics, 1989, New Age International Limited, [1] (頁面存檔備份,存於互聯網檔案館), page 7.
- ^ Brzychczy, Stanisaw; Gorniewicz, Lech. Continuous and discrete models of neural systems in infinite-dimensional abstract spaces. Neurocomputing. 2011, 74 (17): 2711-2715. doi:10.1016/j.neucom.2010.11.005.
- ^ Griva, Igor; Nash, Stephen; Sofer, Ariela. Linear and nonlinear optimization 2nd. Philadelphia: Society for Industrial and Applied Mathematics. 2009: 7 [2024-02-10]. ISBN 978-0-89871-661-0. OCLC 236082842. (原始內容存檔於2020-06-16) (英語).
- ^ 6.0 6.1 Dekking, Frederik Michel; Kraaikamp, Cornelis; Lopuhaä, Hendrik Paul; Meester, Ludolf Erwin. A Modern Introduction to Probability and Statistics. Springer Texts in Statistics. 2005. ISBN 978-1-85233-896-1. ISSN 1431-875X. doi:10.1007/1-84628-168-7 (英語).
- ^ Poyton, A. A.; Varziri, Mohammad Saeed; McAuley, Kimberley B.; MclellanPat James, Pat James; Ramsay, James O. Parameter estimation in continuous-time dynamic models using principal differential analysis. Computers & Chemical Engineering. 2006-02-15, 30 (4): 698-708. doi:10.1016/j.compchemeng.2005.11.008.
- ^ Odifreddi, Piergiorgio. Classical Recursion Theory: The Theory of Functions and Sets of Natural Numbers. North Holland Publishing Company. 1992-02-18: 18. ISBN 978-0444894830.
- ^ van Douwen, Eric. Handbook of Set-Theoretic Topology. North Holland: Elsevier. 1984: 113-167. ISBN 978-0-444-86580-9.
- ^ Clogg, Clifford C.; Shockey, James W. Handbook of Multivariate Experimental Psychology. Boston, Massachusetts: Springer Publishing Company. 1988: 337-365. ISBN 978-1-4613-0893-5.
- ^ Thyagarajan, K.S. Introduction to Digital Signal Processing Using MATLAB with Application to Digital Communications 1. Springer Publishing Company. 2019: 21-63. ISBN 978-3319760285.
- ^ Miller, Jerry L.L.; Erickson, Maynard L. On Dummy Variable Regression Analysis. Sociological Methods & Research. 1974-05, 2 (4): 395-519. doi:10.1177/004912417400200402.
- ^ Hardy, Melissa A. Regression with Dummy Variables (Quantitative Applications in the Social Sciences) 1st. Newbury Park: Sage Publications, Inc. 1993-02-25: v. ISBN 0803951280.
- ^ Olkin, Ingram; Tate, Robert. Multivariate Correlation Models with Mixed Discrete and Continuous Variables. The Annals of Mathematical Statistics. 1961-06, 32 (2): 448-465. doi:10.1214/aoms/1177705052 .
- ^ Fitzmaurice, Garrett M.; Laird, Nan M. Regression Models for Mixed Discrete and Continuous Responses with Potentially Missing Values. Biometrics. 1997-03, 53 (1): 110-122. doi:10.2307/2533101.
- ^ Sharma, Shalendra D. On a Continuous/Discrete Time Queueing System with Arrivals in Batches of Variable Size and Correlated Departures. Journal of Applied Probability. 1975-03, 12 (1): 115-129. doi:10.2307/3212413.